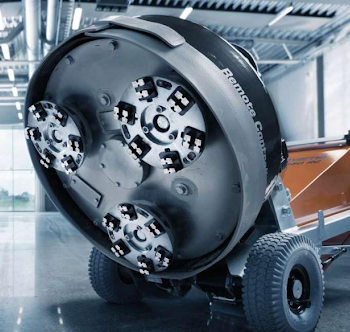
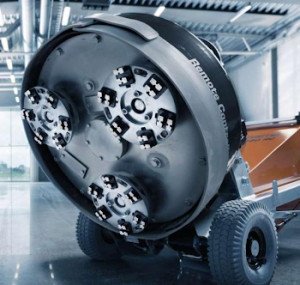
Leverage AI for intelligent predictive maintenance in manufacturing processes
Summary
A key part of smart manufacturing and a modern factory approach involves real-time monitoring of machinery operating conditions. AI models can automate monitoring tasks, resulting in reduced downtime and improved production quality. Using deep learning to quickly triage malfunctioning or defective machinery can result in significant cost and production efficiencies.
In this particular use case, Peltarion experts developed a solution for a multinational enterprise with over 10K employees to detect when their floor grinders were wearing down, based on audio data.
Objectives
Machine failure and defects result in lost business. In fact, equipment maintenance expenses alone make up between 15-40% of a manufacturer’s total production costs. Current maintenance approaches often feature blunt techniques such as blind checks or are centered around machine usage alone.
Without specific and reliable data on machinery operating conditions, manufacturers tend to be cautious and undergo more maintenance than is necessary, leading to excessive downtime and lost production.
Even though most manufacturers continue to favor preventative maintenance approaches, AI-enabled predictive maintenance is significantly more cost effective and efficient, resulting in savings of 30-40% and a tenfold return on investment. These benefits are substantial, especially given that equipment which fails before its expected lifetime can lead to more severe consequences, including significant downtime and costs that are over 10 times as much as a maintenance program.
By request from the multinational enterprise, Peltarion was tasked to solve two dilemmas:
- The detection of malfunctions in the machinery were done manually through audio cues from the floor grinders and required several years of experience.
- The company had no data or way of recording when their machines started to wear down.
Solution
Every piece of equipment has an estimated lifespan that depends on numerous factors like usage and material. Continuous equipment monitoring results in a high volume of data that can be laborious and costly for a human operator to analyze.
In contrast, deep learning models can handle large volumes of data efficiently, and they can accurately identify defects and anomalies with much greater speed and consistency, saving time and money.
For example, it could learn to identify the sounds of a malfunctioning machine or spot failing equipment based on sensor data.
In this case, Peltarion generated sound data by adding microphones to the floor grinders and created a model to detect when the discs of the machines needed to be changed.
Outcome
As an initial step, the solution allowed the firm to have novice operators detecting when their floor grinders are wearing down and needed to be changed. Ultimately, the firm will incorporate the model into its next generation autonomous floor grindings, removing the need for operators all together.