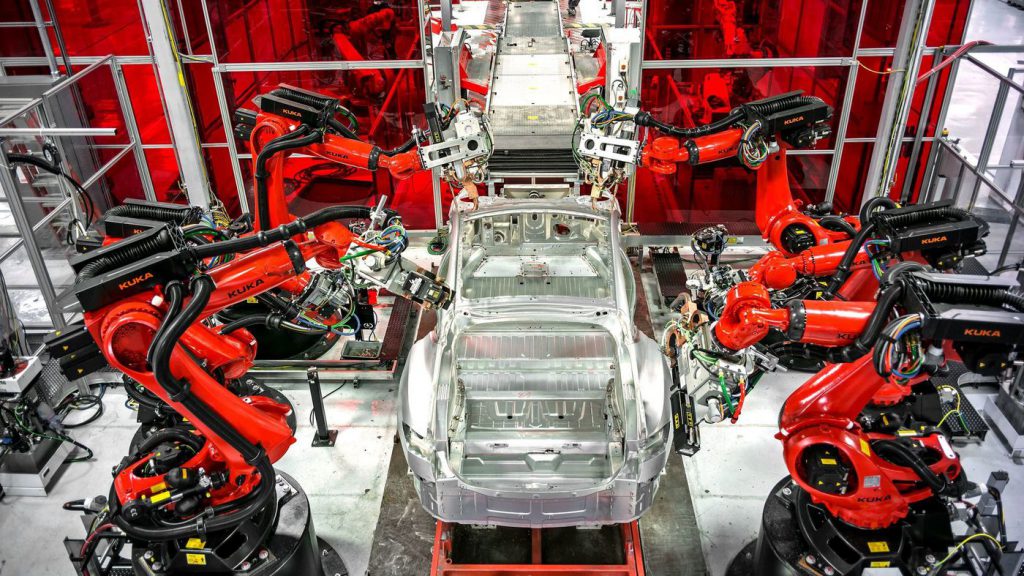
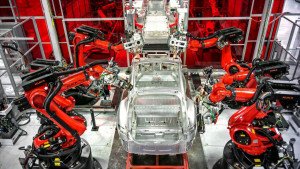
Predictive Maintenance (PdM)
Predictive maintenance (PdM) is conducting maintenance to prevent predicted problems rather than conducting maintenance on a fixed schedule or when an issue arises.
Predictive maintenance techniques and software have evolved over the years. Companies do not need to rely on techniques where they import data to spreadsheets and uncover insights manually. With the increasing usage of AI and machine learning algorithms in predictive maintenance tools, now, businesses can accurately predict maintenance tasks thanks to big data.
Advanced equipment such as Internet of things (IoT) and Industrial Internet of things (IIOT) and robots are generating more data than ever before. However, factories’ analytics capabilities have not caught up to this. According to Fero Labs, manufacturing companies discard 98% of all the data they can collect because they do not have the operational analytics capabilities to integrate that data into their operations.
And are they right to discard that data? No, according to McKinsey&Company. McKinsey predicts $1-4T value creation p.a. in 2025 due to IoT in the factory setting globally. A large portion of that value creation is due to industrial analytics and predictive maintenance. Naturally, we would expect hype around these impressive values and according to GE, even back in 2014, big data analytics was one of top 3 priorities for >84% of CxOs in factory settings.
Predictive maintenance (PdM) and industry 4.0 companies step in to fill the gap between data and insights for industrial companies. Predictive maintenance software allows companies to store and analyze critical outputs of their machinery. One of the key things to do with that data is to improve maintenance and input parameters of their machinery.
Here’s how you implement a predictive maintenance program: There are 2 common ways to roll-out a predictive maintenance solution.
Building an in-house solution leveraging open source libraries like Python. Depending on your company’s skill-set, this could be attractive. If you have engineers willing to spend their time off work to play with sensor data and predict failures, you could end up with a rudimentary predictive maintenance solution for free.
However, in most cases, engineers do not have time for such large side projects and you would need to hire a data scientist. You could even hire a specialized predictive maintenance engineer but this path will probably end up being expensive and slow.
The alternative is buying a predictive maintenance solution that has built-in integrations with your advanced machinery allowing you to get up-to-speed quickly and paying as you reap rewards.
Once your software solution is in place, a bit of organizational work is needed to reap the full rewards. Firstly, all engineering teams should be notified of the advanced analytics capabilities you needed to build for predictive maintenance. For example, difficult-to-measure improvement programs like Six Sigma or Lean need to be monitored with your firm’s new analytics capabilities. Secondly, maintenance teams need to learn to use the full analytical capabilities of the solution. It is not efficient if they continue to do a complete checkup on robots when a slight adjustment on a specific component is needed.
One of the most comprehensive studies on potential of industrial analytics was conducted by McKinsey in 2015 and we used their estimates in showing how much improvement is possible. Their study was based on client studies so we believe it can give you a good idea of the potential:
- 50% reduction in downtime due to equipment failures: Asset failures are costly and stressful. An hour of downtime can impact millions in revenues for a $100 M+ company. Since issues can be predicted in advanced, downtime can be minimized. Increasing up-time is a significant challenge for manufacturing or logistics companies with machines that depend on one another. An example is downtime in cranes. As Predikto CEO Mario Montag mentioned, ports experience 800 to a thousand hours a year in downtime due to crane malfunctions which is extremely costly for port operators.
- 3-5% increased machine useful life: Since predictive maintenance reduces machine breakdowns and ensures operation in optimum settings, it can improve machine/robot useful life.
- Reduced environmental impact: As machines remain useful for longer periods and as their efficiency increase with advanced analytics, companies will waste less natural resources. Predictive maintenance is one of the few initiatives that both help companies bottom line and their corporate social responsibility goals.
- 10-40% reduction in maintenance costs: Since planned maintenance is based on a schedule, there will be cases when maintenance tasks will be performed when they are not needed. Predictive maintenance can prevent such inefficiencies. Furthermore, predictive maintenance systems inform technicians about the changes they need to do to the system based on symptoms. For example, let’s assume that sensors show increased vibration is observed in a machine. If there’s a strong correlation between malfunction of a specific part and increased vibration then technicians can focus first on the possibly malfunctioning part, completing only necessary maintenance activities, saving time.
- 10-25% reduction in worker injuries: Leveraging sensor data with analytic systems will help industries find new ways to avoid injuries. Reduced breakdowns and accident avoidance systems that can alert or even halt equipment when there is a danger to a worker, can dramatically improve factory conditions and minimize worker injuries.
- 10-20% reduced waste: Sub-optimal operation that is not detected, can result in wasteful production. Raw material, energy, labor costs and machine time get wasted in such instances. Predictive maintenance systems can uncover issues that can result in waste before they arise.
- Advanced analytics: Setting up predictive maintenance involves collecting sensor data from diverse machinery. Once that data starts to be automatically collected, analysts have a trove of information ready for analysis. This data can be used to identify parameter and process optimization opportunities.
- Improved product quality and increased customer satisfaction: Detailed sensor data and ability to observe results of interventions create a virtuous cycle of experimentation and learning. As teams adjust machine parameters and improve results, they uncover means to improve quality.
- Increased employee morale: Downtimes, operation with sub-optimal parameters not only impact output but also impact employee morale. It is stressful to rush to solve problems when they arise. Predictive maintenance minimizes such instances.
- Improved performance over time: Predictive maintenance systems are learning systems. They implicitly create a knowledge base of issues and understand their root causes based on feedback from technicians or sensors on the shop floor.
2021-05-20 10:25
Image
|