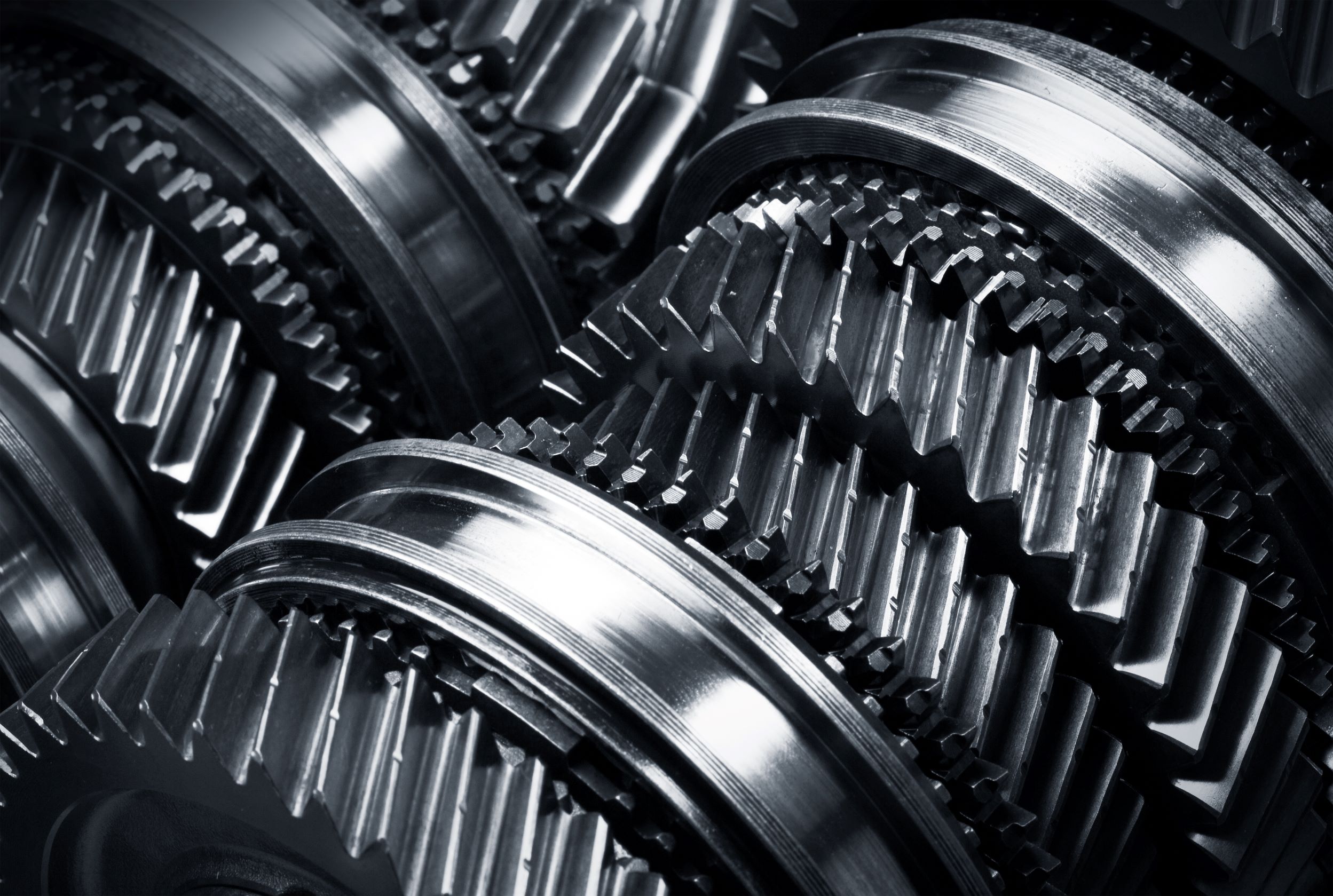
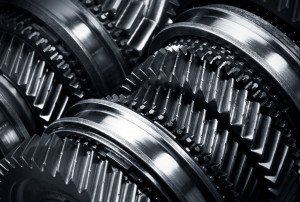
Early detection of gearbox failure
Based on historic vibration data collected by sensors in a gearbox shaft, MultiViz Vibration detected asset failure two months before it happened.
Its Automatic Mode Identification found a new operational mode in the equipment, caused by inner raceway failure in the high-speed shaft bearing, which ultimately led to the replacement of the entire gearbox.
The objective was to use data collected from vibration sensors in a gearbox to detect asset failure in a way that could allow the performance of predictive maintenance in the equipment, avoiding the high costs associated with a unplanned stop in the machine.
Data was collected from an accelerometer located in the axial direction of a shaft connecting a gearbox to a power generator. The vibration signals were recorded every 12 hours for 50 months since the machine began operation. Each segment was 1.28 seconds long with a sampling rate of 12.8 kHz.
The Mode Identification feature is powered by Viking Analytics' AMI unsupervised algorithm for multivariate time series. It performs multidimensional time series data segmentation and clustering in vibration data, detecting time periods in which the data exhibits a similar structure and reflecting them into operational modes.
Operational modes often correctly capture typical states of an asset, like on/off, different production settings or developing failures. The emerging of a new, previously not seen mode, can point towards either an asset being used in a new way or a failure.
MultiViz Vibration first detected an operational mode at the beginning of the life of the machine, identified as a normal operational mode. However, after 11 months, a new operational mode emerged, lasting for three months. The new mode was caused by a high-speed shaft bearing fault, which had only been caught by traditional maintenance techniques two months after the data first identified it.