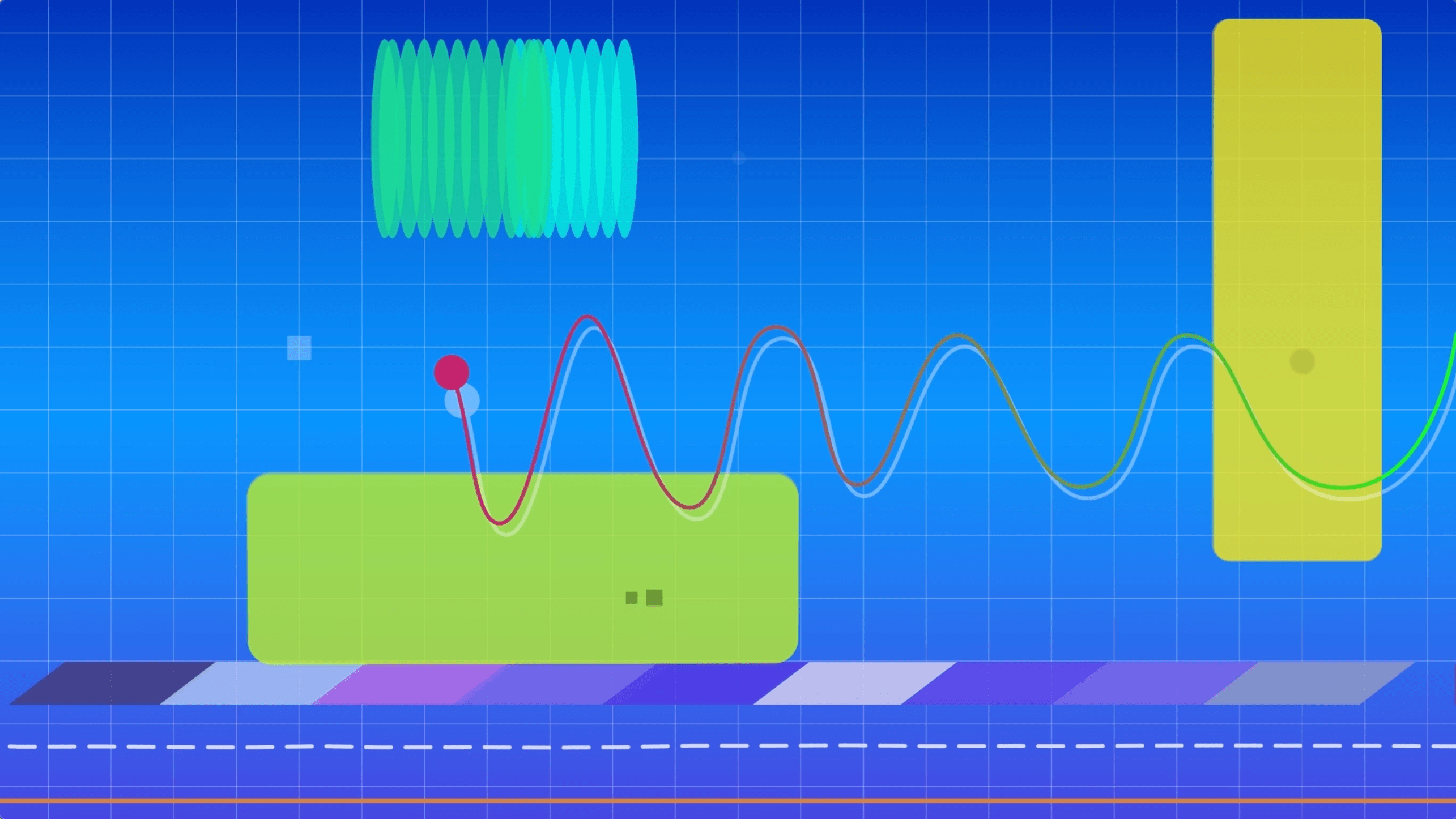
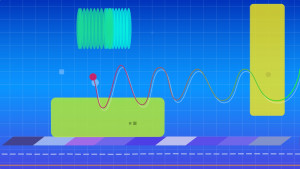
LEARN HOW TO MAXIMIZE THE VALUE OF YOUR DATA ASSETS FOR AI
Even the most powerful AI model, when fed with poor-quality data, is outperformed by a weaker AI model trained on high-quality data.
This contemporary saying circulating in the tech world emphasizes the significance of data quality in AI development. While cutting-edge AI models are integral for producing impressive outcomes, they aren’t the only crucial factor. Data serves as the critical fuel that drives AI models and its quality fundamentally determines their effectiveness. Regardless of a model’s superiority, it is only as good as the data it’s fed.
Since the establishment of our company Oxide AI, we have actively prioritized source data. Our laser-focused dedication has led us to develop proprietary AI and data representation technology, designed for the precise task of harvesting vast quantities of source data in the global financial sector. Our commitment to data management excellence has enabled Oxide’s lean team to make a significant impact and take comprehensive control over the data. This allows us to leverage the potential of AI automation for scaling, rather than relying on manpower. Most rewarding of all, our solid data practices pave the way for limitless AI application possibilities; the well-managed and organized data can easily be transformed and packaged for varying use cases.
Oxide’s approach is based on a deep understanding of several essential elements of source data. We start by examining data’s origin and establishing its authenticity. Following that, we also assess its intrinsic value and evaluate the sufficiency of its representation and validation. Finally, we consider the different metrics that can effectively quantify it. We only move forward with developing and training AI models once we’ve done such a thorough analysis.
To guide you in thinking more strategically about your data in the context of AI, we offer a few key insights grounded in multiple years practical industrial experience:
Data Veracity: assessing quality, accuracy, and authority is challenging and time-consuming for large datasets. The key is tracing the data generation process, especially if it involves human input, which often introduces errors. If a validation process exists, it’s critical to deeply comprehend it.
Data Structuring: transforming unstructured data into a structured format is often highly beneficial. This is particularly true when unstructured data, such as customer interactions, detailed product descriptions, or internal documents, can enrich structured datasets. Such data is invaluable for training modern statistical machine learning models, like large language models (LLMs), and can be harnessed in chatbots or advanced search systems.
Volume and Velocity: large data volumes demand significant engineering resources, so evaluate the value of data against the incurred costs. Accumulating data without a clear purpose is inefficient. When dealing with high-velocity data, consider real-time AI-transformation models to capitalize on the data’s immediate value.
Temporal Data: timestamps on data creation, modification, and storage can unlock potential future value. It’s advisable to initially preserve data in its raw form before database insertion. This approach preserves the capacity to replay historical data for new storage methods or AI refinement without loss of information.
Uniqueness and Information Decay: unique data is inherently more valuable. Even widely accessible data can gain value when intelligently linked with multiple sources. Recognize that information value degrades over time; prioritizing the utility of data at different intervals can optimize its usefulness.
Watch our video on this topic: