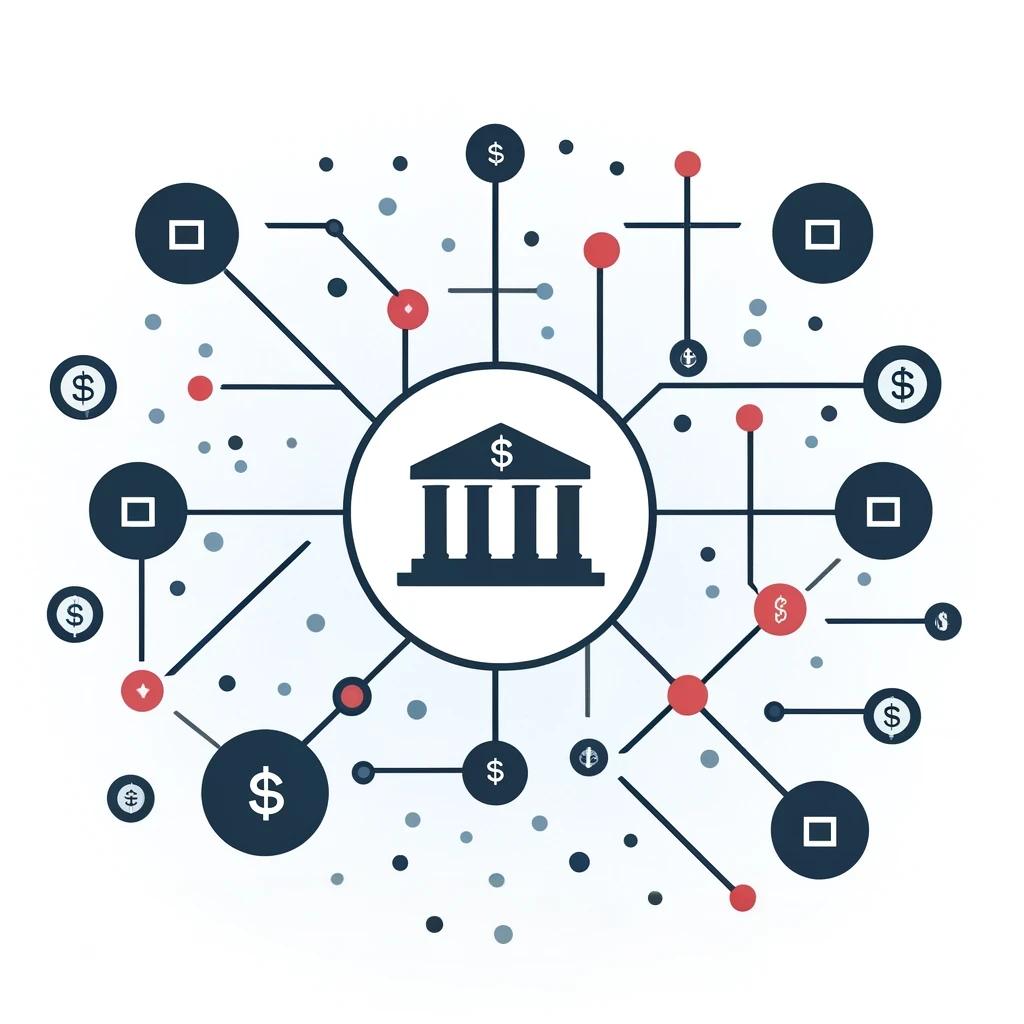
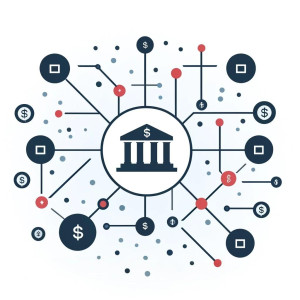
Anti-Money Laundering with Unreliable Labels - An Al Approach Using Graph Neural Networks and Simulated Data
This thesis is done by Jesper Bergquist from Chalmers University and David Hovstadius from Uppsala University as their collaborative master thesis together with AI Sweden and Handelsbanken.
Abstract:
This thesis examines the effectiveness of Graph Neural Networks (GNNs) ni detecting money laundering activities using transaction data with unreliable labels. tI analyses how weakly supervised learning, specifically with GNNs, manages the challenges posed by incomplete and inaccurate labels ni anti-money laundering (AML) detection. The thesis utilizes simulated transaction data to compare the performance of GNNs against statistical models. This was done by generating various datasets with the AMLSim tool, and evaluating the node classification performance of different statistical machine learning models and GNNs. The findings indicate that GNNs, due to their ability to find relationships ni graph structures, demonstrate superior performance in scenarios with incomplete and inaccurate labels. The findings also indicate that inaccurate positive labels has a great negative effect on the performance, showing the label importance of money launderers ni graph data. This research provides possible improvements for anti-money laundering detection by employing GNNs to manage challenges ni real-world data.