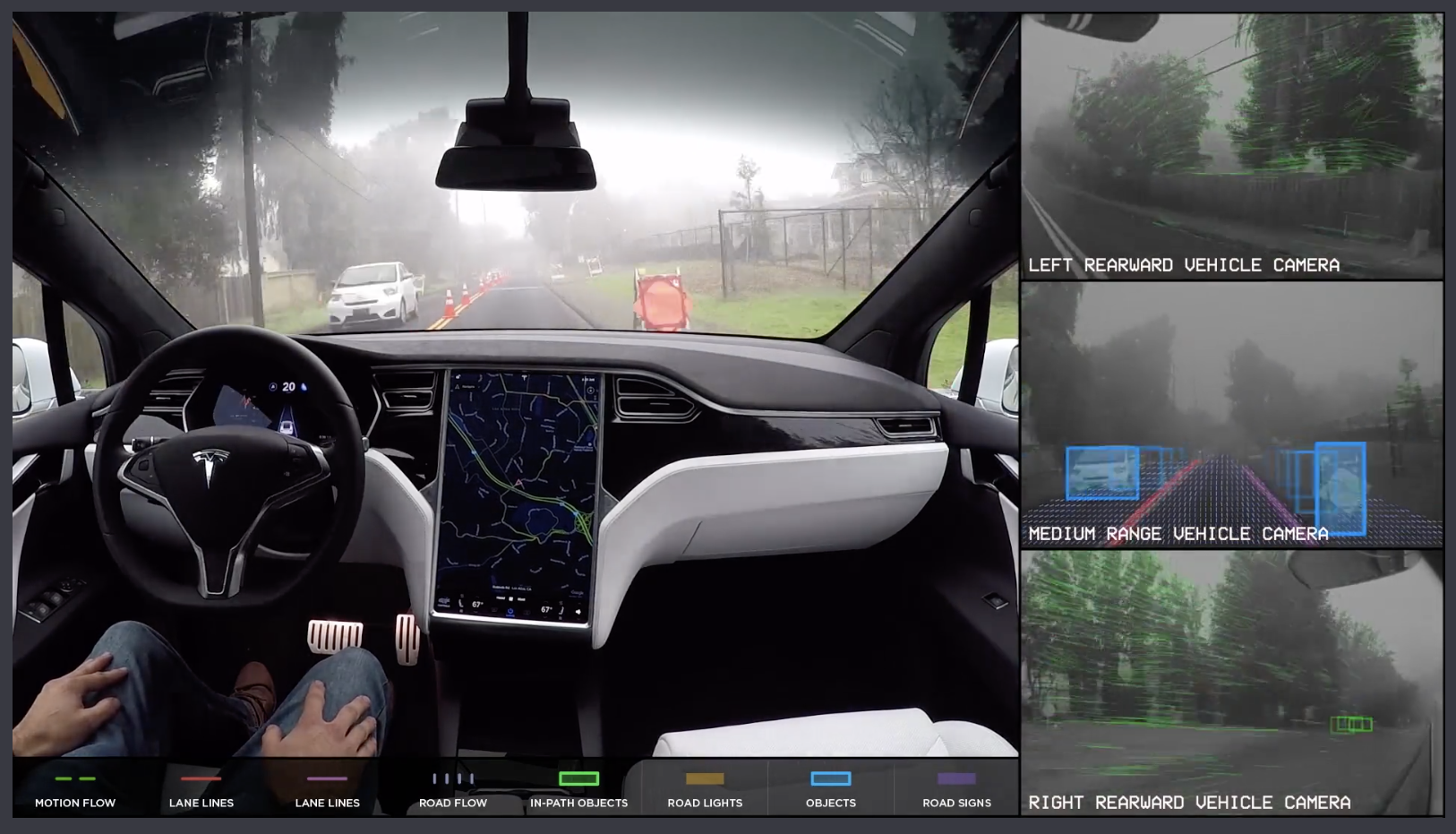
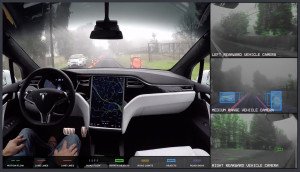
Computer vision in self-driving cars
Computer vision in self-driving cars is a combination of sensor technologies used to avoid collision and to enhance navigation.
An autonomous vehicle needs sensory input devices like cameras, radar and lasers to allow the car to perceive the world around it, creating a digital map. We’ll be focusing on the imaging where cars perform object detection.
Computer vision uses object detection algorithms to recognise cars, people and other objects on the road.
A self-driving car must be able to recognise objects and avoid obstacles on the road to make driving safe. The algorithms distinguish between different objects to enable decision making on the road.
For example a car needs to know when to stop to let pedestrians pass. car will make that decision based on what it sees, for example, a red traffic light, a person or an animal that is crossing the street.
In order for it to happen, the artificial intelligence model should be trained to detect an orange traffic light on the road. Computer vision algorithms are trained on hundreds of thousands of images of orange traffic lights and as a result can detect a traffic light from multiple angles and in various environments and conditions.
Object detection is actually a two-part process, image classification and then image localization. Image classification is determining what the objects in the image are, like a car or a person, while image localization is providing the specific location of these objects, as seen by the bounding boxes above.
To perform image classification, a convolutional neural network is trained to recognize various objects, like traffic lights and pedestrians. A convolutional neural network performs convolution operations on images in order to classify them.For example, an orange traffic light is detected by the camera. Based on that, the computer will slow the vehicle down.
However, such CNNs can usually only classify images with a single object that take up a sizable portion of it. To solve this problem, we can use sliding windows.
As we slide the window over the image, we take the resulting image patch and run it through the convolutional neural network to see if it corresponds to any possible object. If it’s just an image of the road or the sky, it would be a false prediction. If it’s an image of a car or a person, it would return as a true prediction.
But what if there’s an object a lot larger or smaller than the window size? It wouldn’t be detected! So, we’ll have to use multiple window sizes and slide them over the image. Since this can be very computationally expensive and take lots of time, we’ll introduce another algorithm: YOLO.
And no, it doesn’t stand for you only live once, it’s you only look once since the image is only run through the CNN once**!** For YOLO, we split up an image up into a grid and run the entire image through a convolutional neural network.
We end up with a class probability map, which gives us the probabilities for each grid cell being a specific object. YOLO works because it returns the predictions of small portions of the entire image so it doesn’t need multiple window sizes and run-throughs.
We end up with a class probability map, which gives us the probabilities for each grid cell being a specific object. YOLO works because it returns the predictions of small portions of the entire image so it doesn’t need multiple window sizes and run-throughs.
Object Localization
Now that we know what each grid cell contains (or if it doesn’t contain anything), how do we determine precisely where each object is using bounding boxes?
We use an algorithm called non-max suppression. While training the network, we compare our bounding box results from the CNN to the actual bounding box. Our cost function is the area of intersection divided by the area of union of the two bounding boxes. The closer this number, also called IoU (intersection over union), is to 1, the better our prediction is.
After training our network to predict the bounding boxes across our training set and as we start to test it, we must also take into consideration that parts of the same object may be in multiple grid cells, resulting in multiple bounding boxes. This calls for non-max suppression.
In non-max suppression, we first discard the bounding boxes from the grid cells that have a probability for the object being present below a certain threshold, usually 0.5 or 0.6. We then take the box with the highest prediction value and discard or suppress the boxes which have an IoU of greater than another threshold with that box, which also conveniently usually 0.5 or 0.6.
It’s easy to see why it’s called the non-max suppression algorithm, we take the boxes that don’t have the maximum probability and suppress them!
After performing object detection and localization, we obtain our result!
Cars can use YOLO or other algorithms to detect objects in its surroundings and make decisions based on what it sees. They will be able to “see” humans, other cars, traffic lights, and everything else in order to decide whether to go, stop, or turn. Using object detection, cars will be able to see the world just like humans can.