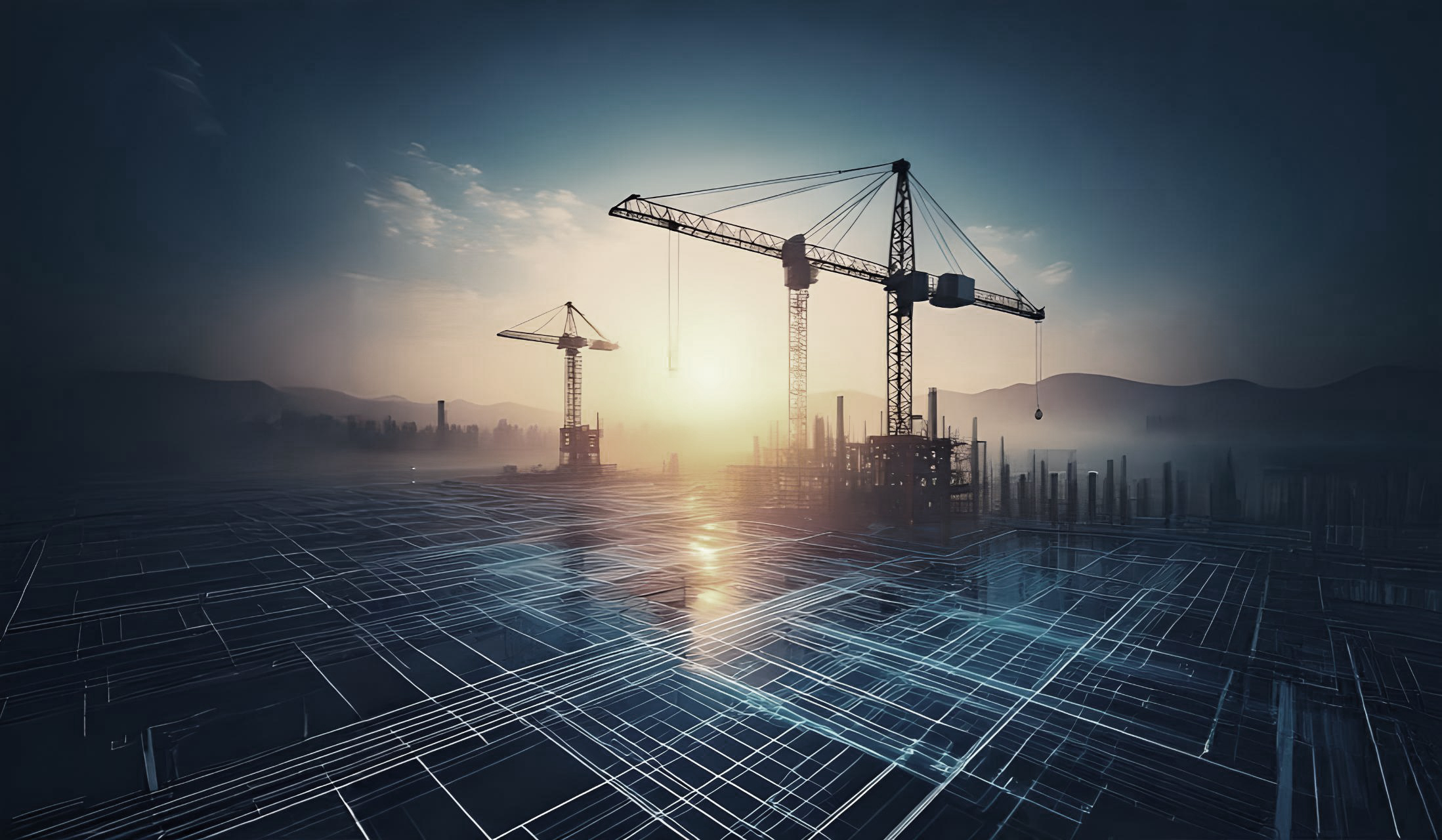
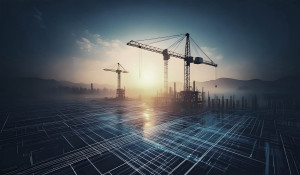
Tendering in the Swedish construction industry is in large parts based on AMA (Allmän material- och arbetsbeskrivning). This means, that in many cases, tenders submitted for construction projects overlap to some extent, whenever a tender request includes the same requirements as earlier requests.
Construction enterprise NCC wanted to investigate the possibility of using an NLP tool for analysing tenders they have submitted in the past and build a database to be used for comparing past tenders and new tender requests. Tender request contents could then be mapped to requirements in new requests, based on the AMA codes so often used in these tendering processes, and a similarity ratio for sections under the same AMA headings could be extracted. Doing so would save time and lessen the effort needed for manually studying vast numbers of past tenders, looking for identical or similar past requirements.
Apart from drawings, textual descriptions of buildings make up most tender request documents, and the idea is to use analyses of such texts and compare them to other tender requests to be able to judge to what extent they overlap.
The goal of the project was to build a database based on a limited number of past tenders and to use this dataset to investigate whether an NLP tool can be used for identifying text on AMA code level, to find a similar past tenders and for making time estimates, that have historically been created from scratch for each new tender.
By mapping tendering documents to the database, it should be possible to find and cross-reference standard AMA sections and identify project-specific text, leading to less manual work and facilitate data-driven decisions.
A database to use for testing was built using 10 past tender documents. This data was then used for analysing tender requests in pdf format, to find sections that were identical or similar. The tool provided data that was used for creating dashboards for displaying likenesses between past and current tender requests, with a very high level of granularity.
The NLP prototype built proved to be very efficient in analysing large text sections, accurately identifying similarities and differences, with the ability of the dashboard tool to visualise very detailed information on the likeness between the texts compared. With shorter texts, however, the tool was sometimes fooled by misspellings and word omissions.
One other major takeaway from the project was that it would be necessary to use a construction-specific dictionary to reach greater accuracy—the dictionary used for this prototype contained general-type Swedish words.
The project also concluded that a tool like this will also need a high level of standardisation in regard to the outcomes of past tenders and how data is collected, stored and compared between projects. Only then will an NLP analysis tool for tenders in the construction industry be as accurate as it can potentially become.