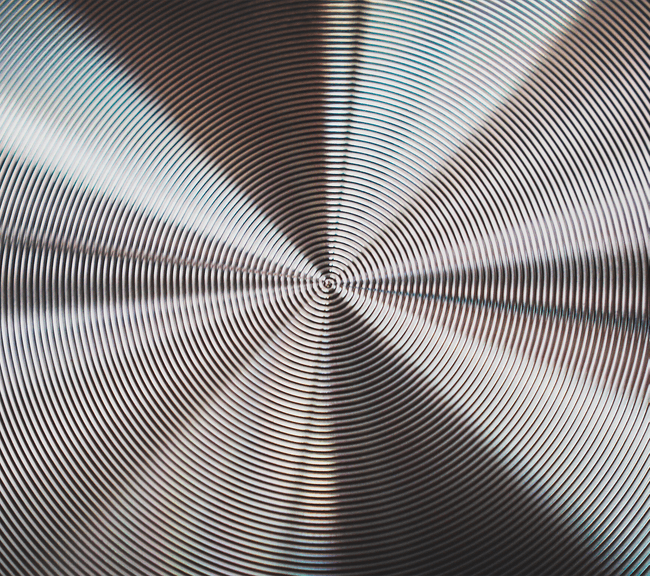
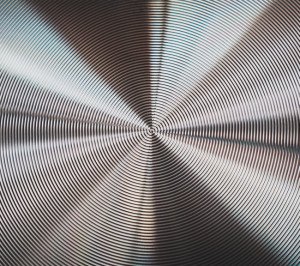
Using AI for complex quality assurance within Industrial 3D printing
Our partner Amexci specializes in this type of Industrial 3D printing through a process called selective laser melting (or L-PBF). Recently, Peltarion has been working with Amexci to create a type of model that enables the company to break new ground when it comes to quality control, both speeding up how quickly the technology can be applied to industrial settings and also setting new standards for the type of quality control that can be done.
When using and fusing metals through the process of L-PBF, the high temperatures and the nature of the materials means that a product might deform or overheat in unexpected ways, which creates an added need for process control. When using traditional metal manufacturing methods like molding, defect detection is usually limited to surface detection, but when fusing multiple thin layers on top of each other like in Industrial 3D printing a new opportunity to capture a set of images and sensor data for each individual layer arises.
This data can then be used to identify potential faults in each layer. The problem up to this point has been that, because of the many layers at which this data is captured, each product generates thousands of images, making it highly ineffective for a human to go through them. As a result, many Industrial 3D printing companies now hold vast amounts of data that they simply can’t afford to analyze.
Having thought about this problem for a while, Amexci contacted Peltarion to see if possible to build a model around this.
Trying to reduce the complexity of the proof of concept we decided to start with data from just one type of 3D printer using aluminium. After making the appropriate quality assurance on the dataset, the remaining dataset contained just 24 defective products (but which amounted to over 20,000 images). Despite the limited datasets with only 24 faulty products the team was able to build a clustering tool to demonstrate that there was a high likelihood that a reliable model could be built with enough data. The clustering was based on the feature representations from a trained image auto encoder, meaning that the clustering will group similar images.
After getting a strong indication that we were onto something meaningful for the industry, the next step has been to collect more data to train a high-performance model that can be integrated into the production process.
To move forward with this, Amexci has now launched an initiative called the Rosetta Protocol, where companies within the Industrial 3D printing industry are able to offer their image data to enable further training of the model, and receive predictions from the Deep Learning model on what potential process deviations were captured in return.
The industry response has far exceeded expectations, indicating that this is exactly the type of initiative that people have been wanting to see.
2021-11-19 20:13
Image
|