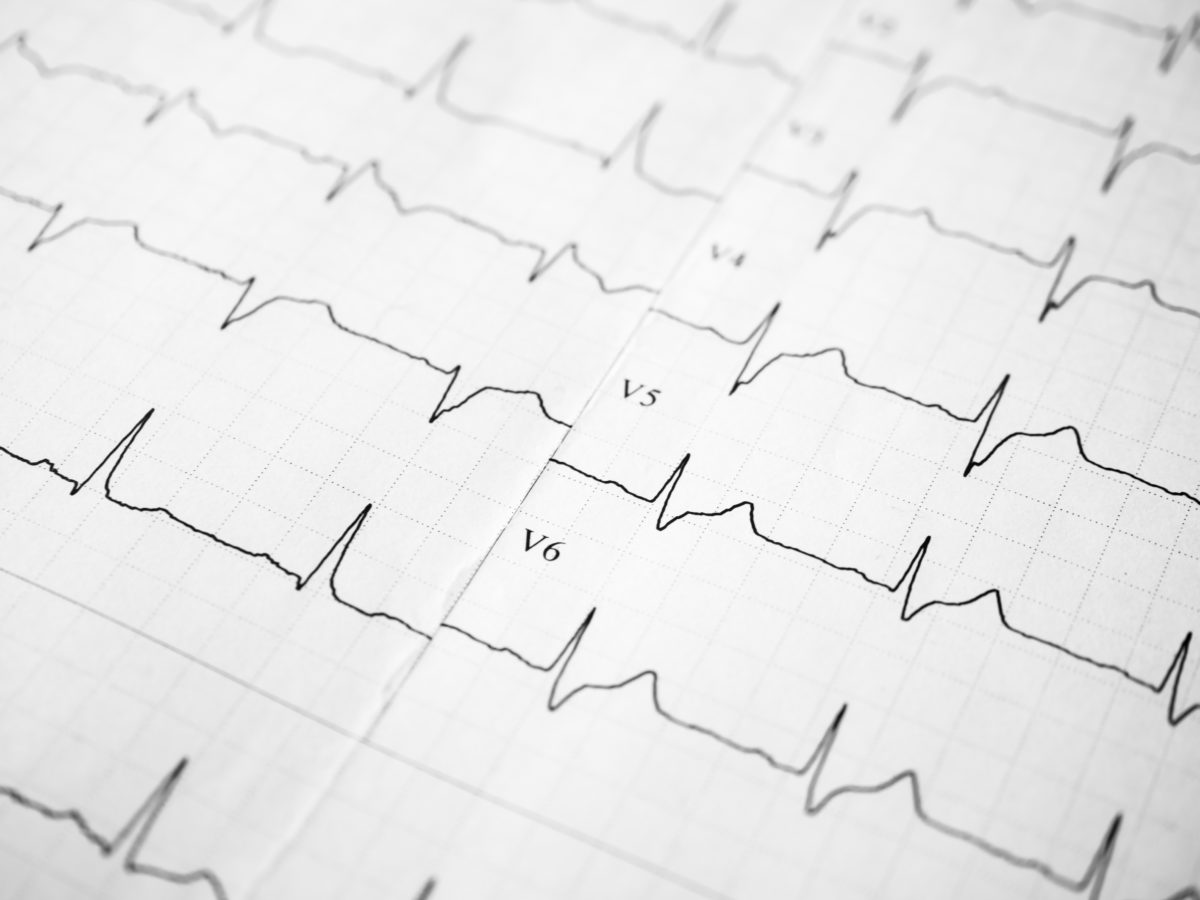
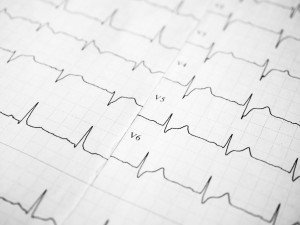
Predicting underlying paroxysmal atrial fibrillation using raw electrocardiogram time-series data
Modulai worked together with the client and leading cardiologists in creation of a model to predict paroxysmal atrial fibrillation.
Every day, thousands of people suffer from stroke due to an underlying undiagnosed atrial fibrillation (AF) condition. Stroke often results in severe conditions such as paralysis or, in many cases, even death. For this reason, precise and early diagnosis of AF is an essential part of stroke prevention.
Zenicor Medical Systems AB has become one of the leading Medtech companies in Europe in the fields of early diagnosis of arrhythmias and stroke prevention for health care. They have developed a unique system, Zenicor-ECG, for the early detection and diagnosis of arrhythmias easily and cost-effectively.
Working together with the client and leading cardiologists at Karolinska Institutet as domain experts, the Modulai team tested different and modified versions of state-of-the-art CNN architectures for raw time series data. Modulai was able to create a model to detect underlying AF in normal-looking ECGs.
To further gain insight into the model, explainability methods were employed to find out how the model was able to predict the condition. The model was wrapped in a Flask API and dockerized as a last step.
Modulai has together with Zenicor Medical Systems AB trained a deep learning model to predict an underlying paroxysmal AF condition in patients, using single-lead electrocardiogram (ECG) time series of the patients’ sinus ECG as input. The project was funded in part by Vinnova.