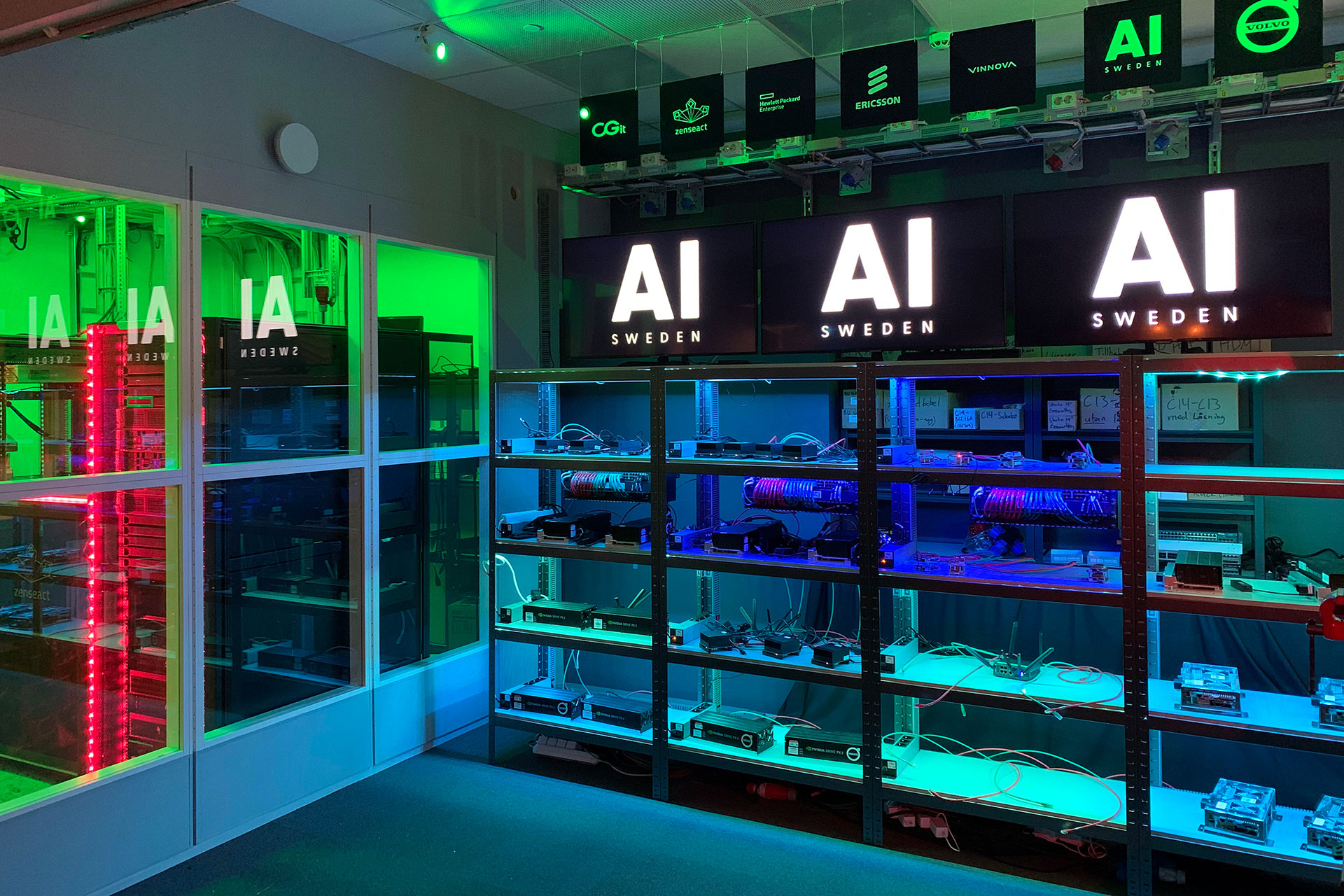
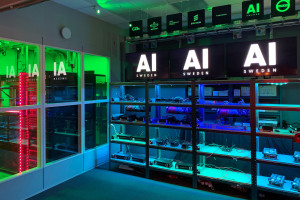
Addressing Privacy Threats, Intellectual Property Risks, and Data Re-Identification
As artificial intelligence (AI) technology evolves, protecting privacy and intellectual property is becoming increasingly vital. AI systems handle vast amounts of sensitive data and valuable innovations, making them prime targets for security threats. Key concerns include unauthorized access to confidential information, theft of AI models, and the re-identification of anonymized data. These risks can significantly impact both the security and value of AI systems.
Why does it matter?
Privacy and intellectual property threats are critical because they affect the confidentiality and ownership of data and technological advancements. Unauthorized exposure of personal or proprietary information can lead to serious consequences, including financial loss, identity theft, and reputational damage. Ensuring robust protection against these threats is essential for maintaining trust and safeguarding competitive advantages.
What are privacy and intellectual property threats?
Privacy threats involve unauthorized access to or disclosure of personal data, potentially leading to various forms of misuse. For example, AI systems handling sensitive health or financial data are at risk if this information is not adequately protected. Intellectual property threats relate to the theft or unauthorized use of proprietary technologies, designs, and algorithms. These threats can undermine a company’s competitive edge and the value of its innovations.
What is model stealing and training data exfiltration?
Model stealing refers to the unauthorized extraction of an AI model’s details, allowing attackers to replicate or exploit it without permission. Techniques such as querying the model to infer its underlying structure or behavior can lead to model theft. This compromises the original model’s competitive advantage and intellectual property value.
Training data exfiltration involves the unauthorized extraction of data used to train an AI system. Since training data often contains valuable and sensitive information, its unauthorized access can reveal proprietary insights or expose confidential details. For example, if attackers access a financial institution’s training data, they might uncover sensitive customer information or discern business patterns for malicious purposes.
What is re-identification of anonymized data?
Re-identification is the process of linking anonymized data back to individuals it originally represented. Although anonymization aims to protect privacy by removing personal identifiers, sophisticated techniques can sometimes reverse this process, revealing identities. For instance, when anonymized health data is combined with other datasets, such as demographic or geographic information, it can potentially lead to the re-identification of individuals.
Protecting against these threats
To mitigate privacy and intellectual property threats, organizations should implement comprehensive data protection strategies. This includes encryption, strict access controls, and continuous monitoring to safeguard data and AI models. Legal and ethical practices, such as advanced data anonymization and secure data handling protocols, are crucial for protecting privacy and intellectual property.
Contact us if you are a partner in AI Sweden and want to learn more or engage with AI Sweden in AI security.
→ Sign up here to receive updates from the AI Security Newsletter!
Related projects at AI Sweden
LeakPro: Leakage Profiling and Risk Oversight for Machine Learning Models https://www.ai.se/en/project/leakpro-leakage-profiling-and-risk-oversight-machine-learning-models - Focuses on identifying and mitigating information leakage in machine learning models.
Federated Fleet Learning https://www.ai.se/en/project/federated-fleet-learning - Explores decentralized approaches to AI model training to enhance privacy.
Federated Learning in Banking https://www.ai.se/en/project/federated-learning-banking - Investigates federated learning techniques for securing sensitive financial data.
July 29, 2024 by Madeleine Xia